Artificial Intelligence in the Financial Sector – Regulatory Challenges Between DORA, MiCA, the AI Act, and Banking Supervision
Have you got a question?
I. Introduction
Artificial Intelligence (“AI”) is rapidly gaining traction in the financial sector, with applications ranging from fraud prevention and customer service to automated decision-making. While AI offers significant potential for efficiency and innovation, financial institutions face a complex interplay of new and existing regulatory requirements. In navigating between the EU Artificial Intelligence Act (AI Act), the Digital Operational Resilience Act (DORA), the Markets in Crypto-Assets Regulation (MiCA), and traditional banking supervision frameworks (e.g., MaRisk, EBA Guidelines, KWG), institutions must deploy AI systems in a legally compliant and risk-conscious manner. This article provides an overview of the current regulatory landscape, outlines practical challenges, and highlights intersections in AI risk management.
A. Regulatory Landscape: AI Act, DORA, MiCA, and Banking Supervision
-
1. EU Artificial Intelligence Act The European Union adopted the world's first comprehensive legal framework for AI in late 2023. Regulation (EU) 2024/1689 establishing harmonized rules on artificial intelligence (the "AI Act") entered into force on 1 August 2024, with most provisions applicable from 2 August 2026. The AI Act employs a risk-based approach, prohibiting certain unacceptable practices (e.g., manipulative or discriminatory AI), imposing strict obligations on high-risk systems, and setting minimal requirements for lower-risk categories. Of particular relevance to financial institutions is the classification of credit scoring systems as high-risk, subjecting them to stringent requirements regarding risk management, data quality, documentation, human oversight, and transparency. Such systems must undergo a conformity assessment prior to deployment or market access (CE marking). Breaches of the AI Act may incur fines of up to €35 million or 7% of global annual turnover. The AI Act complements rather than replaces sector-specific rules and leaves room for stricter national labor protections. Member States may assign supervisory responsibility for AI either to sectoral financial regulators or new AI-specific authorities, raising the possibility of dual oversight—particularly for AI-based credit models. Experts have therefore called for close inter-agency coordination to prevent regulatory duplication or conflicts.
-
2. Digital Operational Resilience Act (DORA) The Regulation (EU) 2022/2554 of the European Parliament and of the Council of 14 December 2022 on digital operational resilience for the financial sector (“DORA”) establishes a harmonized EU-wide framework for the digital operational resilience of financial entities. Applicable to banks, insurers, investment firms, and a broad range of financial service providers, DORA consolidates requirements related to IT risk management, cyber incident reporting, and operational resilience testing (e.g., penetration testing for large institutions). DORA supersedes prior national regulations and replaces outdated guidelines, such as the EBA’s PSD2 incident reporting framework. DORA mandates comprehensive ICT risk management, including inventories of all IT systems and AI services, rigorous third-party oversight, and robust business continuity planning. Institutions leveraging cloud-based AI services must ensure due diligence, implement exit strategies, and continuously monitor service provider performance. Even for outsourced AI functionalities, financial institutions retain accountability for associated risks and must evaluate any subcontracting arrangements. Overall, DORA compels institutions to demonstrably reinforce the resilience of their digital—and AI-driven—operations.
-
3. Markets in Crypto-Assets Regulation (MiCA) The Regulation (EU) 2023/1114 of the European Parliament and of the Council of 31 May 2023 on markets in crypto-assets (“MiCA”) provides the supervisory framework for crypto-assets not otherwise regulated. It gradually introduces licensing and compliance obligations for crypto service providers. AI intersects with MiCA in contexts such as algorithmic trading, robo-advisory platforms, and algorithmic stablecoins. MiCA imposes transparency, disclosure, and authorization requirements on providers (e.g., exchanges, wallet providers), and mandates ongoing oversight. AI-based crypto solutions must comply with both MiCA and the AI Act. For instance, a crypto robo-advisor must adhere to MiCA’s conduct and licensing obligations, while its AI algorithm must meet the AI Act's requirements. Notably, MiCA effectively prohibits fully algorithmic stablecoins lacking sufficient reserves. Institutions must thus ensure that AI-driven crypto innovations operate within a licensed and compliant framework, with regulators - such as Germany’s BaFin in coordination with ESMA - scrutinizing AI's potential to facilitate market abuse or investor risk.
-
4. Banking Supervision Requirements (MaRisk, EBA Guidelines, KWG) Independent of new EU regulations, banks must continue to comply with existing national and European supervisory standards. In Germany, the Minimum Requirements for Risk Management (MaRisk) interpret Section 25a of the Banking Act (KWG) and, in its 2023 update, explicitly addressed AI model governance. Section AT 4.3.5 MaRisk requires banks to assess AI model appropriateness before deployment, ensure ongoing validation, maintain model explainability, and uphold data quality. Institutions must be able to explain AI-based decisions (e.g., credit scoring outcomes) and allow customers the opportunity for review in automated credit rejections. Human intervention must remain possible ("human in the loop"). These requirements apply irrespective of technological sophistication and are complemented by the EBA's outsourcing and ICT risk guidelines. Cloud-based AI service providers are treated as outsourcing partners, requiring comprehensive risk assessments, contractual safeguards, and continuous monitoring. In sum, AI systems must be integrated into a bank’s overall governance and control frameworks, with supervisory authorities increasingly focused on the robustness and transparency of machine learning models.
B. Practical Challenges of AI Use in Financial Institutions
-
1. Anti-Money Laundering (AML) and Know Your Customer (KYC) AI systems are increasingly being deployed to detect suspicious transactions and to generate customer risk profiles. While this promises significant efficiency gains in anti-money laundering (AML) efforts, it also presents notable challenges. A central issue is traceability: supervisory authorities expect that institutions must be able to explain why an AI algorithm classified (or failed to classify) a particular transaction as suspicious. So-called “black box” models encounter significant limitations in this regard. Moreover, institutions must strive to minimize false positives (unjustified alerts) and avoid false negatives (actual money laundering cases that go undetected) - a difficult balancing act when training AI models. Practitioners report that, to date, few institutions have implemented AI comprehensively within AML processes. According to an international survey1, only 18% of compliance teams currently use AI/ML solutions, while 40% have no plans to do so at all. Another barrier is the supervisory stance: the survey indicated that only 51% of regulators actively support the use of AI - down from previous years. Many supervisory authorities take a cautious or even skeptical approach, which can discourage institutions from adopting AI in compliance functions. Nevertheless, AI systems offer clear advantages in AML and Know Your Customer (KYC) procedures, particularly in analyzing large data volumes (e.g., transaction monitoring) and identifying complex patterns. The challenge lies in embedding this technology within the internal control framework in such a way that compliance with anti-money laundering legislation is maintained. For instance, every automated decision - such as the acceptance or rejection of a customer - must be properly documented in accordance with applicable regulations and must remain subject to manual review where necessary.
-
2. Credit Scoring and Lending In the retail and SME lending sectors, AI models are increasingly being employed for creditworthiness assessments and fraud detection. This area presents a direct collision between innovation and regulatory compliance. On one hand, consumer protection laws and the GDPR mandate transparency in automated credit decisions - customers have the right to understand why a given credit score was low. On the other hand, AI algorithms frequently rely on high-dimensional data sets, including alternative data sources, and complex non-linear models (e.g., neural networks), which significantly hinder explainability. From a regulatory standpoint, banks must ensure that AI-based scoring models do not result in discriminatory effects (e.g., indirect disadvantage to specific demographic groups). This obligation arises from the German Equal Treatment Act (AGG) and supervisory requirements issued by BaFin mandating non-discriminatory scoring practices. Technically, eliminating bias in training data is a complex and resource-intensive task. Moreover, model validation remains a persistent challenge. Under MaRisk and relevant EBA Guidelines, internal risk models must be subject to regular performance testing (backtesting) and independent validation. With self-learning AI models that continuously adapt to new data, the question arises as to how a “moving target” can be reliably validated. Institutions often address this by “freezing” machine learning models at fixed intervals for evaluation purposes. Another critical tension lies between competitiveness and standardization: AI may enable significantly more accurate risk assessments, potentially resulting in lower default rates. However, supervisors are increasingly scrutinizing whether banks might use highly complex models to circumvent minimum capital requirements. The EBA has suggested that while AI models may, in principle, be permissible under the Internal Ratings-Based (IRB) approach, institutions must demonstrate that such models do not produce unduly low risk weights and are consistent with the requirements of the AI Act. This remains a legal and supervisory grey area that will only be clarified through regulatory practice and, where appropriate, further guidance.
-
3. Trading Systems and Market Abuse In the capital markets sphere, certain trading departments increasingly rely on AI for predictive modelling, algorithmic trading strategies, or fraud detection (e.g. market abuse surveillance). Algorithmic trading is already subject to the requirements of MiFID II/MiFIR as well as the Market Abuse Regulation (MAR). High-frequency trading firms, for instance, must implement safeguards such as "kill switches" and report their algorithms to the supervisory authorities. While the formal obligations do not change with the deployment of AI—owing to the principle of technology neutrality—practical challenges do arise. For example, AI-driven trading algorithms must undergo rigorous pre-deployment testing to ensure they do not adopt uncontrolled strategies during periods of market stress. The risk that multiple AI systems may act procyclically in volatile markets and thereby exacerbate events such as flash crashes is tangible and is actively addressed by regulators. Institutions must document the data inputs and assumptions underpinning AI systems used in trading, in order to prevent, for example, the improper use of insider information. Should an AI system make trading decisions that result in market manipulation (such as unintended spoofing through pattern exploitation), the institution remains liable. Accordingly, the compliance function is tasked with the continuous monitoring of AI models and must be prepared to intervene if necessary. The obligation to report suspicious orders (Suspicious Transaction and Order Reports, STORs) naturally extends to AI-generated trading activity. Overall, effective compliance with regulatory requirements necessitates close collaboration between quantitative teams, IT, and compliance departments.
-
4. Outsourcing of AI and Cloud Usage Many banks not only develop AI in-house but also obtain AI functionalities as services from specialised providers or large cloud platforms (i.e. AI as a Service). While this can reduce internal IT burdens, it constitutes regulatory outsourcing. The typical challenges associated with outsourcing are amplified in the context of AI. On one hand, contractual arrangements must ensure that the institution retains all necessary information and audit rights—for instance, to review the fairness and stability of AI models. On the other hand, operational resilience becomes critical: if an AI service fails or malfunctions, it must not cripple the institution's operations. DORA requires contingency plans and regular drills to ensure preparedness. Banks must ask themselves: Are fallback solutions in place should the AI service become unavailable (e.g. manual processes or secondary systems)? How rapidly can a switch to an alternative provider be executed (exit strategy), and are such scenarios contractually and operationally planned for? DORA also obliges institutions to assess the risks associated with the AI provider's subcontractors. The service chain of a cloud-based AI system can be complex—for example, where the AI provider relies on hyperscalers. Here, institutions often encounter information asymmetries: not every cloud provider discloses how its AI models are developed or where data is processed. This conflicts with supervisory expectations of full control. Possible solutions include strengthened audit cooperation or certifications: major cloud providers are already working toward DORA compliance and provide clients with compliance reports. Nevertheless, implementing AI in an outsourced structure remains a balancing act between efficiency gains and loss of control. From a legal standpoint, confidentiality and data protection are particularly relevant: where customer rating or transaction data is processed in an AI cloud service, banking secrecy and GDPR obligations must be observed. This often necessitates data anonymisation or pseudonymisation and robust contractual provisions on data sovereignty.
C. Interfaces Between AI Risk Management and Supervisory Governance
The integration of AI systems intersects with numerous governance areas within financial institutions. The traditional “Three Lines of Defence” model – comprising business units, risk control/compliance, and internal audit – must be applied to AI-related processes as well. For every significant AI system, a designated model owner should be appointed who understands the model and manages its risks. Risk management should incorporate AI risks – including model, IT, and reputational risks – into the institution-wide risk inventory. Executive management remains responsible for reflecting the use of AI in business and risk strategies and for ensuring the adequacy of internal controls (pursuant to Section 25a KWG).
New regulatory touchpoints are emerging: banking supervisors (BaFin/ECB) now review AI models during special audits or as part of the SREP process, while from 2026 onwards, dedicated AI supervisory authorities will also monitor compliance with the AI Act. As noted by KPMG, a single AI-based credit model may end up being scrutinised by multiple supervisory bodies. Consistent documentation and governance are therefore essential to present a coherent picture across all reviews. Conflicting requirements – such as differing interpretations of what constitutes sufficient explainability – must be identified and addressed at an early stage.
The technology-neutral nature of many financial supervisory rules is beneficial in this context: robust model risk management in line with MaRisk already satisfies many of the AI Act’s requirements for high-risk AI. Banks should capitalise on such synergies and adapt their existing validation processes to also cover AI-specific criteria (e.g. transparency, bias mitigation). The compliance function should closely monitor regulatory developments, such as relevant EBA guidelines or future interpretive guidance from BaFin on AI regulation. Equally important is coordination with other legal frameworks—such as data protection laws. The AI Act states that it applies alongside the GDPR without undermining its rights. Where AI makes decisions of significant impact on customers, the right to object under Article 22 GDPR may apply. These cross-cutting issues should be managed centrally, ideally through an institution-wide AI risk management framework (e.g. a dedicated AI governance committee or a Chief AI Officer in larger institutions).
Supervisory authorities also expect that corporate culture and governance reflect emerging AI risks – a principle often framed under “AI Ethics.” Institutions would be well advised to adopt internal policies for the responsible use of AI (similar to a Code of Conduct), setting clear expectations for employees and service providers.
-
1. DORA and Operational Resilience in AI Solutions Particular emphasis is placed on the operational resilience of AI-based processes, especially when delivered by third parties or via cloud services. DORA requires that critical failures in digital systems be controlled and that business continuity be promptly restored. For AI solutions, this means that robustness and fail-safe mechanisms must be incorporated from the outset. If, for example, a bank uses cloud-based AI for transaction monitoring, it must define the response in case of a system failure—will all questionable transactions be conservatively blocked, or will fallback rule-based systems be activated? Data resilience is equally critical: AI is only as reliable as the data it consumes. DORA-compliant institutions therefore maintain redundant data infrastructures and monitor data flows. Cloud-based AI systems also require regular emergency drills, simulating scenarios such as service unavailability, to test whether switching to internal systems or alternative providers is feasible. DORA’s requirements for Threat Led Penetration Testing (TLPT) - scenario-based cyberattack simulations—should extend to AI components. For example, institutions should test how AI models respond to adversarial inputs. Are such attacks detectable, and can the institution respond effectively? Model maintenance is another key aspect of resilience: AI models require continuous upkeep (retraining, updates). DORA’s principle of operational continuity means banking operations must not be disrupted by routine AI maintenance. Accordingly, maintenance windows must be planned carefully, and strategies such as blue-green deployments considered to avoid downtime. Supervisors will pay particular attention to single points of failure: if a critical AI system (e.g. for risk management) lacks redundancy, this is viewed as a vulnerability. Institutions must have backups or clearly defined workarounds. The worst-case scenario - an AI system behaving uncontrollably (e.g. a self-learning algorithm generating nonsensical outputs) - must also be addressed. Institutions must implement monitoring alerts and mechanisms to promptly deactivate such systems before harm occurs. In sum, DORA compels institutions to treat AI not merely as an IT project but as an integral component of operational stability -subject to the same rigorous continuity planning as core banking systems.
-
2. Legal Conflicts, Grey Areas, and Outlook Despite substantial progress in regulatory developments, unresolved issues remain at the intersection of AI and financial supervision. A clear area of tension exists between the cross-sectoral AI Act and existing sector-specific rules. For example, a bank’s AI-driven credit scoring model may be certified as a "high-risk AI system" under the AI Act, yet still be rejected by banking supervisors due to deficiencies in validation. It will fall to regulatory practice in the coming years to determine how such discrepancies are resolved. European supervisory authorities have already flagged the need for action. The EBA has emphasised that the AI Act must be clarified for the financial sector to avoid unintended consequences—such as dual regulation or uncertainty over which authority has jurisdiction. This may lead to the issuance of guidelines or technical standards specifically tailored to AI Act implementation within financial services. A conceivable example would be an EBA guide on supervisory expectations for AI models, bridging the gap until the AI Act becomes fully applicable in 2026. Similarly, EU or national standards for AI governance may emerge, analogous to existing IT governance frameworks. BaFin, for its part, laid initial groundwork with its 2021 principles paper and the 2023 MaRisk update, though further development is expected. Another area of concern is liability and accountability in the use of AI. The regulatory position is clear: the institution remains responsible, even where decisions are made automatically or via external AI systems. Nonetheless, practical questions persist - who is liable if an AI algorithm systematically makes erroneous credit decisions or if a trading bot causes substantial losses? In such cases, civil liability principles (e.g. organisational fault, potential product liability on the part of the AI developer) will apply—a field where legal norms remain underdeveloped. Grey areas also exist with emerging AI technologies such as generative AI (e.g. ChatGPT), which are increasingly used in customer interaction or internal processes (e.g. code generation, reporting). These raise concerns regarding data protection (e.g. input of sensitive data into external systems), compliance (e.g. monitoring outputs to avoid misleading content), and intellectual property rights in AI-generated materials. The EU is currently drafting a Code of Practice for generative AI, expected in 2025 - financial institutions will likely need to align with its principles to demonstrate good practice. From a supervisory perspective, the ECB has already made it clear that it will focus on AI risks within the context of digital transformation. In its supervisory priorities for 2025–2027, the ECB underscores the need for a structured, risk-based approach to AI adoption in banking and calls on institutions to adapt their digital strategies accordingly. The ECB expects banks to adequately mitigate risks arising from AI and cloud use and to implement best practices. This indicates that supervisory reviews and possibly future guidelines on machine learning in risk management will follow. BaFin is also likely to refine its administrative practices - with circulars or guidance documents expected once the AI Act becomes fully applicable - to clarify overlaps with national obligations.
II. Concluding Remarks
AI in the financial sector is evolving within an increasingly dense regulatory framework. For legal and compliance professionals, this requires staying abreast of emerging rules and bridging the gap between cutting-edge technology and rigorous legal compliance. The challenge lies in implementing AI systems that deliver operational benefits while remaining auditable, controllable, and – where necessary – constrainable.
Given the high regulatory expectations (and the potential for significant penalties), financial institutions should proactively establish internal safeguards and foster cross-functional collaboration between IT, business units, compliance, and legal departments to achieve “AI compliance by design.” The years leading up to 2026 will be pivotal in implementing the new regulatory mandates. Institutions that now invest in sound AI risk management will not only gain the trust of regulators and customers but also secure a competitive advantage in a financial ecosystem that values responsible AI use.
Book a call back
Share this article
Got a question?
Please complete this form to send an enquiry. Your message will be sent to one member of our team.
Related posts
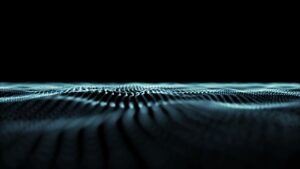
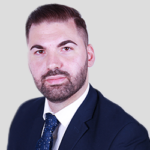
Successful Settlement for Injured Client Secured by Oracle Solicitors
Personal injuries sustained as a result of being involved in an accident can be traumatic and life-altering experiences for the injured parties. Whether
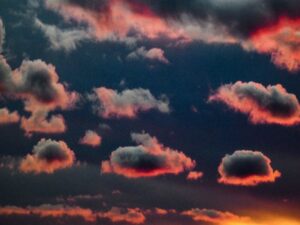
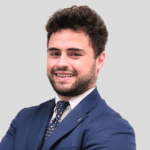
Cars: The EU grants three years to manufacturers to meet emission targets
The recent announcement by the European Union regarding a three-year extension for meeting the emission targets for internal combustion engine vehicles has reignited
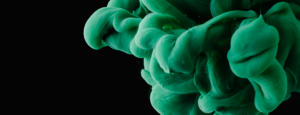
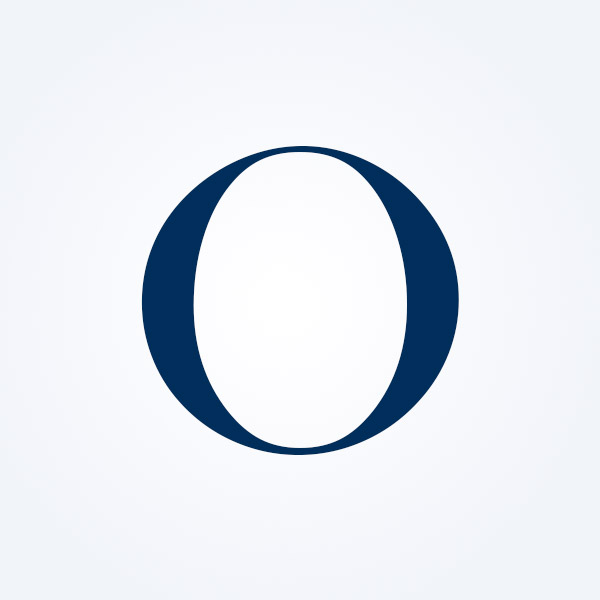
Failure to Prevent Fraud: How Prepared is Your Organisation?
Introduction In a decisive move to combat corporate fraud, the UK government has enacted the Economic Crime and Corporate Transparency Act 2023 (the
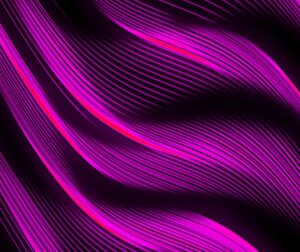
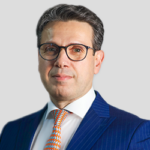
Legal Harmonization of Intellectual Property Rights in Europe: Balancing National Sovereignty and Regional Integration
The protection of intellectual property (IP) rights in Europe has evolved into a cornerstone of the region’s knowledge-based economy. Despite substantial progress through